How Does Traditional Marketing Mix Modeling (MMM) Work?
Jan 2, 2025
Whether you call it marketing mix modeling or media mix modeling (don’t worry – we’ll get into the difference), MMM is often positioned as a solution for marketers who want to understand the effectiveness of their marketing spend.
Great idea, right? But understanding that "effectiveness" is a difficult nut to crack:
- Consumers engage in many behaviors that are hard to measure – online and offline.
- Buyers are influenced by a wide variety of factors, both known and unknown.
- Brands can accidentally wind up measuring correlation instead of causation – someone clicked on an ad, but maybe they were going to buy regardless of the ad. How do we know?
- Web and mobile tech are increasingly curtailing advertiser measurement – and the threat of third-party cookie deprecation remains.
In-platform reporting and click-based attribution are two ways that marketers often measure this today. But those solutions have their own problems:
- Reported conversions are often double-counted across platforms, leading to overstatement of credit.
- A click-driven methodology misrepresents channel contribution. For example, it doesn’t take into account the impact of an ad someone may have viewed and not clicked, even if they ended up converting because of the ad.
Traditional MMM – whether software you purchase or an in-house solution – tries to solve this problem through a model that illustrates correlations between consumer activity and channel spending over time. When it works, conventional MMM can:
- Recommend media spend and optimization across channels. For example, if you’re deploying marketing budget across more than one channel, a model can provide guidance (again, based on correlation, not causation) on the optimal spend level for each channel.
- Answer "what if?” questions and forecast business outcomes: "If we increased online spend by 20% next month, the model forecasts a 15% increase in sales."
- Model other types of changes, like pricing or promotional strategies: "What happens if we offer a 10% discount in-store? The model predicts a 5% boost in sales.”
So: What’s the hangup? Nothing, inherently – if all you care about is correlation. Let’s get into it.
A note on media mix modeling vs. marketing mix modeling
These two terms are often used interchangeably. In practice, you can make this distinction:
- “Media mix modeling” refers specifically to analyzing and optimizing media spend across channels (ex: TV, online, radio)
- “Marketing mix modeling” takes a broader approach, incorporating all marketing efforts, including pricing, promotions, and other external factors that influence sales.
How traditional MMM works
You can break down building a traditional MMM into these foundational steps:
1) Data collection.
This includes data from across all promotional platforms, such as TV, online advertising, and in-store activities. In addition to marketing data, external factors like seasonality, economic trends, or competitive actions can be collected to enrich the dataset and calibrate more accurate modeling. Collection requires setting up infrastructure to receive and store data.
2) Data infrastructure and preparation
Data has to be clean, high-quality, and standardized to foster consistency over long periods. For example, this could involve correcting missing values, removing duplicates, and harmonizing different data formats and sources.
3) Feature exploration and selection
Once the data is ready, the next step is to explore and identify relevant variables (features) to include in the model.
This involves determining which marketing channels, external factors, and business trends influence performance metrics like sales. Variables might include TV spend, online spend, in-store spend, seasonality, and time trends, among others.
4) Model building
With the data and features squared away, the relationships between marketing efforts (inputs) and business outcomes (outputs) are represented mathematically. MMM is often based on linear regression, but more advanced techniques, such as machine learning (ML) models, can make it easier to capture non-linear relationships or interactions between variables.
5) Model evaluation
Once the model is built, its performance must be rigorously tested. Statistical metrics can help determine how well the model explains past results and predicts future performance.
An example of a traditional MMM
Enter: RetailCo. RetailCo is a brand that spends on TV ads, online ads (Google and Facebook), and in-store promotions. They want to determine the contribution of each channel to sales and understand where they should increase or decrease spending.
RetailCo first collects sales data over the past 12 months, together with monthly spend on TV ads, online ads, and in-store promotions. (Note: in practice, brands will typically want at least 24 months worth of data.)
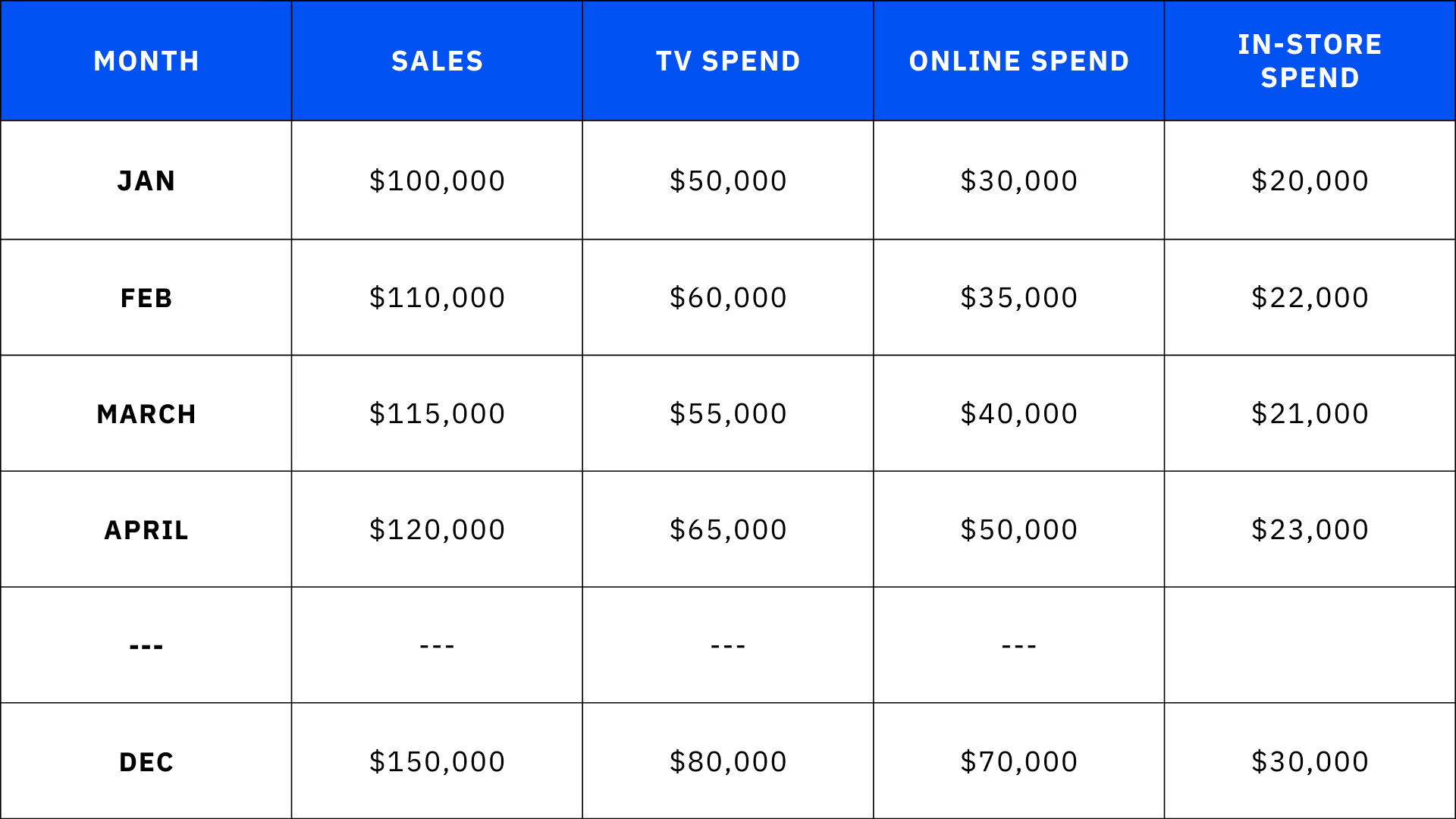
Next, they build a model.
It may sound easy, but this requires significant expertise and coding to build – RetailCo might hire a team who can write Python or R to create this model. This is a resource-intensive step that generally requires several experts to develop, test, and iterate over time.
At the end of the analysis, RetailCo ends up with an equation that includes a coefficient for each input. This coefficient (essentially a multiplier) represents how strongly an input is associated with an outcome – like sales.
It might look like this:

Based on the coefficients, RetailCo could conclude that:
- TVsSpend, with a coefficient of 0.8, is less effective compared to Online spend.
- Online spend is the most efficient channel. For every $1 spent, they get 1.2 units of sales.
- In-store spend is the least effective channel.
Based on this model, they can reallocate spend levels to market more efficiently.
The resource-intensive reality of traditional MMM
MMM requires significant historical data – at least two years’ worth – to produce meaningful results. Brands don’t just have to gather the data, but also ensure that it’s consistent, complete, and accurate.
Building and maintaining a model often requires building and managing the infrastructure in-house, which demands a dedicated team. A typical team includes 8-10+ specialists such as data scientists, analysts, engineers, and product managers, all with prior experience in this domain.
Even with the right team, achieving the same accuracy, precision, and speed as specialized third-party solutions can be tough. Businesses often spend 3-6 months just hiring and mobilizing this team, and 12-24 months iterating to optimize testing practices and processes.
Traditional MMM only gets you so far
Traditional MMM is built on correlational data, not causal relationships. And while it can help explain what happened (note: past tense) and identify trends, it doesn’t explain what specific tactics caused specific outcomes.
Much of marketing measurement relies on correlation, which can be misleading. Brands need a way to establish causation, and that means incrementality testing to determine if a decision causes an outcome.
Consider these 2 scenarios:
- Correlation: Your Sunday routine involves taking your kids to get ice cream. You see a billboard on your way that advertises ice cream from your favorite ice cream shop. It’s a cute ad, but you would have bought ice cream from this shop regardless. The billboard did not cause you to buy ice cream.
- Causation: You’re running errands with your kids, and see a new billboard for an ice cream shop. The billboard makes you think you should surprise your kids with some ice cream, and so you go to that shop and buy some. The billboard caused you to buy ice cream.
Ad platforms like Meta and Google are exceptional at targeting people who are already intending to make a purchase. Their algorithms are optimized to deliver ads to the right people at the right moment. However, this doesn’t necessarily mean the ad caused the purchase – it was simply there in the face of existing intent.
Traditional MMM measures correlation. Brands need to understand causation – or might as well be lighting money on fire.
To measure causation, we use incrementality experiments. By creating control groups (who are not exposed to a treatment) and treatment groups (who are), we can establish a counterfactual: what would have happened if the marketing activity had not occurred?
This experimental approach allows brands to isolate and measure the true lift driven by concentrated marketing tactics, offering a clear understanding of incremental impact.
Using incrementality experiments together with traditional MMM
Traditional MMM and incrementality experiments can be used together such that each tool reinforces the other – it's called a Causal MMM. With this tool, experiments can actually be used to calibrate models and improve precision using causal factors.
Traditional MMMs are generally noisy – no matter if they're SaaS or homegrown. Because they rely on observational data, the incrementality read from the traditional MMM will be uncertain, and sometimes very uncertain depending on how much signal is in the data. Additionally, since traditional MMM is so flexible, there can be multiple different sets of parameter estimates that all fit the data equally well.
Experiments can be used to both increase precision of the model estimates as well as to help “rule out” plausible parameter sets that don’t match the incrementality tests.
Using MMM to identify possible changes in ground truth can help to structure the experimentation roadmap. You might imagine someone saying, “The MMM is indicating that Pinterest performance has improved since we released new creative. Let’s prioritize a geo test in that channel next month.”
Or, you might notice that one channel’s incrementality estimate from the MMM conflicts with data you’re seeing in your digital-tracking or last-touch model. That is a great channel to go and test!
Combining all of the information from tests and trends can be challenging, but MMMs aren’t reliable unless they’re powered by incrementality experiments – that’s the bottom line. Traditional MMMs illustrate correlations, while incrementality experiments illustrate causality. Until they’re unified in one, pairing them is your best bet for sound and trustworthy decision-making.