Beyond the Buzzwords: Why Transparency Matters in Incrementality Testing
Apr 29, 2025
Precision. Accuracy. Causality. If you’re shopping around for measurement solutions, you’ve probably seen these words – or variations on these themes – come up time and time again.
We get asked all the time about what makes us different from fill-in-whatever-measurement-vendors-you’re-currently-evaluating. And that’s good! You’re a responsible buyer, and we want to be responsible partners. It might sound hard to believe, but our team buys SaaS solutions, too – and we know the feeling of seeing all the right buzzwords but not knowing who or what to believe, or why it matters, or how the vendor can prove it.
We can talk about our science until the cows come home – in fact, we like to. But you might not be a scientist, or an economist, or a causal inference expert. So let’s try something different and instead share a bit more about the transparency of our science and why it matters.
Transparency becomes especially critical in two very distinct phases of experimentation:
Before you launch
Imagine you’re gearing up to run an experiment on a channel where you invest millions each year, but you still aren’t sure how much it really moves the needle. You partner with an incrementality vendor (or try to design the test yourself) and anxiously await the results… only to learn the test was underpowered and delivered no clear insights. In other words, you poured hundreds of thousands of dollars into a test that shrugged its shoulders, left you with an inconclusive outcome, and cost you weeks of precious time.
At Haus, we put power and precision (aka standard errors) front and center. Before any customer comes on board, we run a feasibility study to confirm that their test will be sufficiently powered. If the study doesn’t meet necessary requirements, we won’t bring that brand on – period. Then we run thousands of placebo tests to validate the power and precision of the brand’s experiment design. The scars our own team bears from our days before Haus has taught us that unclear test setups waste budget and momentum, and no one should have to endure that. With Haus, you’ll know your test is solid before you ever hit “launch.”
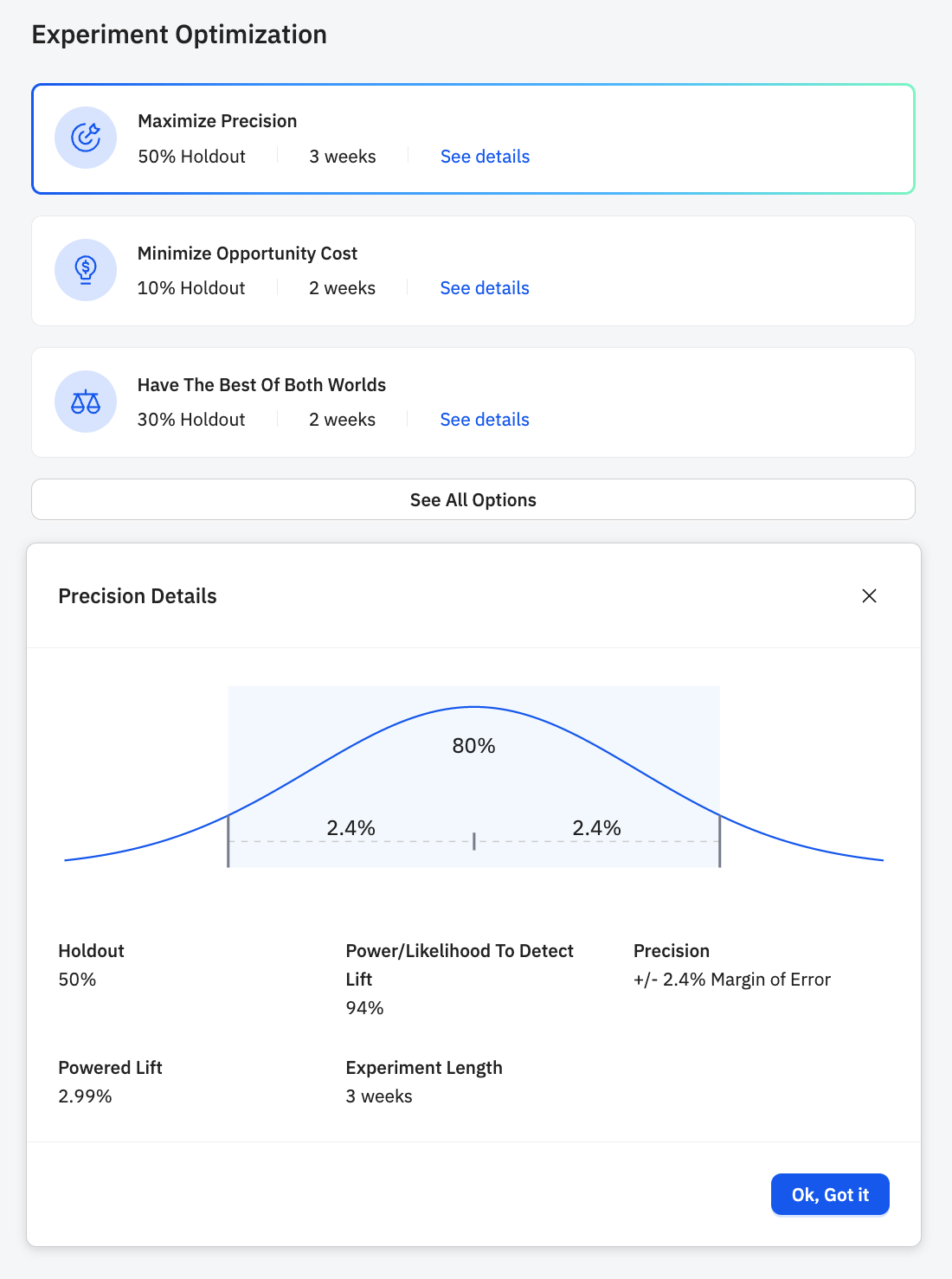
Interpreting and acting on the results
Haus is transparent about estimated uncertainty – a funny concept that can be awkward to wrap your head around, but is really, really important. Being honest and clear about estimated uncertainty helps you know how much to bet on any given result.
Whenever we report a metric from your experiment (e.g., a CPIA of $112), that number is actually a point estimate, meaning it represents the most likely outcome based on the data we have. But every point estimate has a range around it, showing how much it could realistically swing. In Haus, every experiment analysis has probability calculator that informs you exactly how likely your experiment is to hit your target.
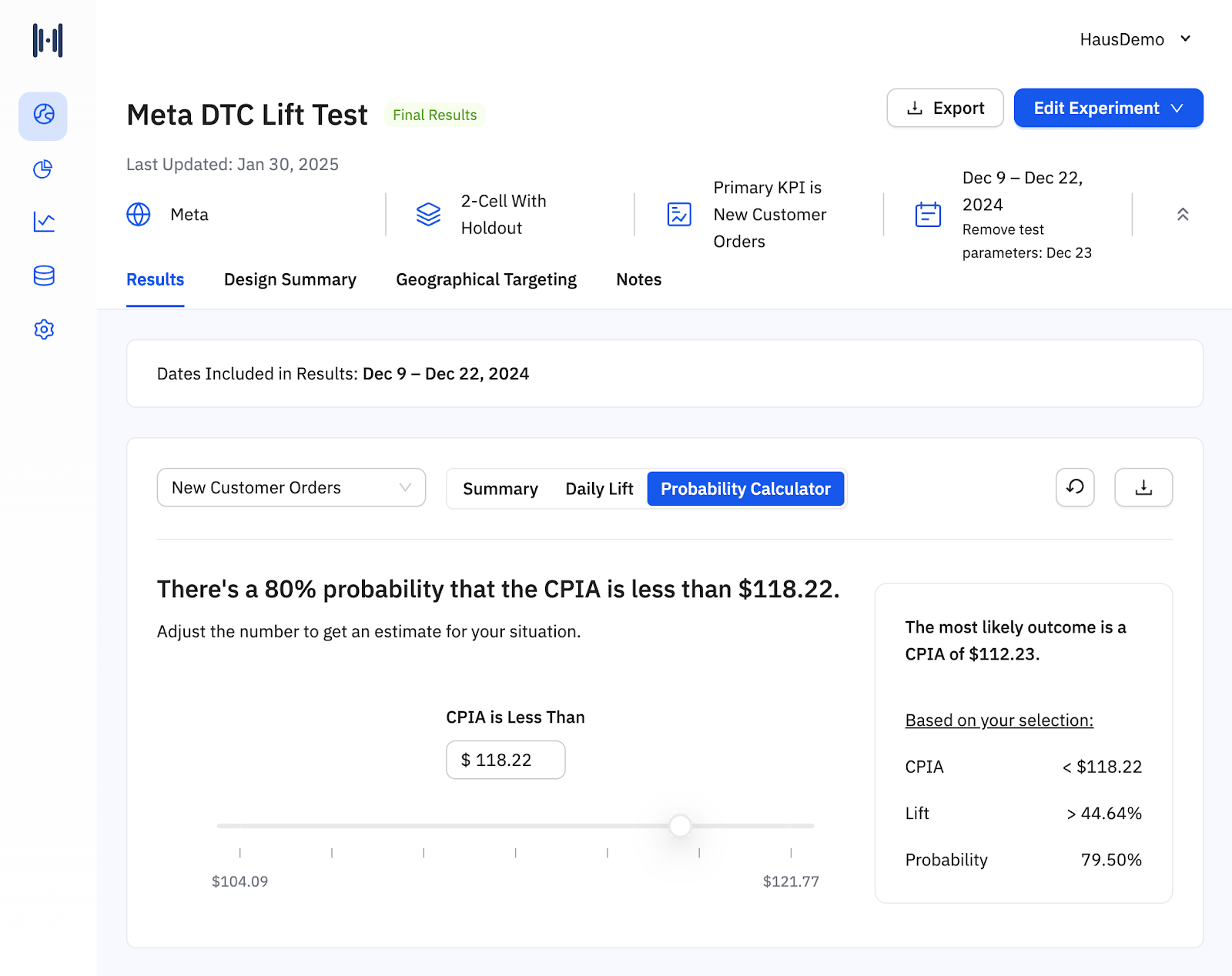
By laying out that range up front, you’ll know exactly how much confidence to place in any given result. Vague confidence scores or precision ‘green-lights’ aren’t specific or reliable; they’re marketing jargon that leaves you flying blind.
The promise of incrementality is that you can bet your business on it. Bad test readouts based on bogus precision claims are potentially no different from measurement alternatives like MTA or traditional MMM where you’re advised to take results with a grain of salt – which is so not the point of incrementality testing. Precision at any point of time – but especially tough times – isn’t a luxury; it’s a necessity. Paid marketing is often the single largest cost center in the P&L, and brands need to have complete information to make responsible decisions like their company depends on it.
Marketing measurement is tough: The Haus industry survey indicates it’s one of the hardest things brands are trying to figure out right now. And it can be expensive, both in dollars and in decision-making time. But it can be made a lot easier (and less costly) with a decent incrementality partner who genuinely believes in the value of transparency around accuracy, precision, and decision-making. Transparent peace of mind, if you will.
From design to analysis
Haus prioritizes honesty and transparency in incrementality testing.
%203.png)
%203.png)
From design to analysis
Haus prioritizes honesty and transparency in incrementality testing.
%203.png)
%203.png)