Would You Bet Your Budget on That? The Case for Honest Marketing Measurement
Olivia Kory, Head of Strategy | Simeon Minard, PhD Economist
Feb 14, 2025
Trust.
It’s what we all want in our measurement solution, right? Trust in the team we’re working with, trust in the methodology behind the experiments, trust in the results we’re analyzing, and trust in the business actions we’re taking as a result.
But how can you know? As privacy regulations evolve, as incrementality testing becomes buzzier, as wallets tighten, it can be tempting to problem-solve marketing measurement with a solution that dazzles you with claims of guaranteed levels of confidence and — wait for it — precision. Who wouldn’t want that?
At Haus, we prefer to think about things a little differently. Transparency – not guaranteed promises — is key. Let us explain.
If you were a betting person, would you bet on this?
Consider this: A friend offers you a tip on a stock that they believe will go up in value by 50% in the next year. They’re so certain that the result will only vary by 2%, meaning that annual returns from purchasing this stock will fall between +48% and 52%.
If your friend is right, it’d be a life-changing decision to put your life savings in this stock. But while your friend may have good reasons to believe the stock will go up, there are an enormous number of factors that affect a stock’s price, and their certainty that it will only vary by 2% is pretty darn unlikely, let alone unreasonable.
Most people wouldn’t trust a recommendation like this — risking their whole life savings — and you can spot this kind of “false certainty” or “false precision” from a mile away. It’ll only vary plus or minus two percent? Yeah, right. Wishful thinking.
You can sniff it out pretty quickly. But when it comes to geo testing, this kind of false certainty in results can be harder to spot — after all, you want to believe in the possibilities. You want to believe the experts you’re partnering with know what they’re doing and will guide you appropriately. And yet time after time, we run into brands who’ve been burned by the false precision and lack-of-transparency siren.
Uncertainty is not the enemy
Repeat after us: Uncertainty is not the enemy. One more time. Uncertainty is not the enemy. Deep breaths.
There is tremendous potential cost to working with a measurement partner that is "guaranteeing" a level of precision. It’s clear as day in the stock-picking example, but not always obvious in other contexts. Even in our own research, we try to stress that while we take great pride in our synthetic control methodology outperforming matched market tests, we can only show that it’s more precise — not that it’s guaranteeing a level of precision. Because it’s not. Every brand has a different level of random noise in their data.
To demonstrate the potential costs of going all in a misleading probability, we did something that scientists do pretty often: created a simulation. Specifically, we simulated KPI data for a hypothetical customer, complete with all sorts of noise – variables that move your business metrics but have nothing to do with your marketing campaign.
Why is this useful? It allows us to see what decision-making might look like under different scenarios, and quantify the risks and costs.
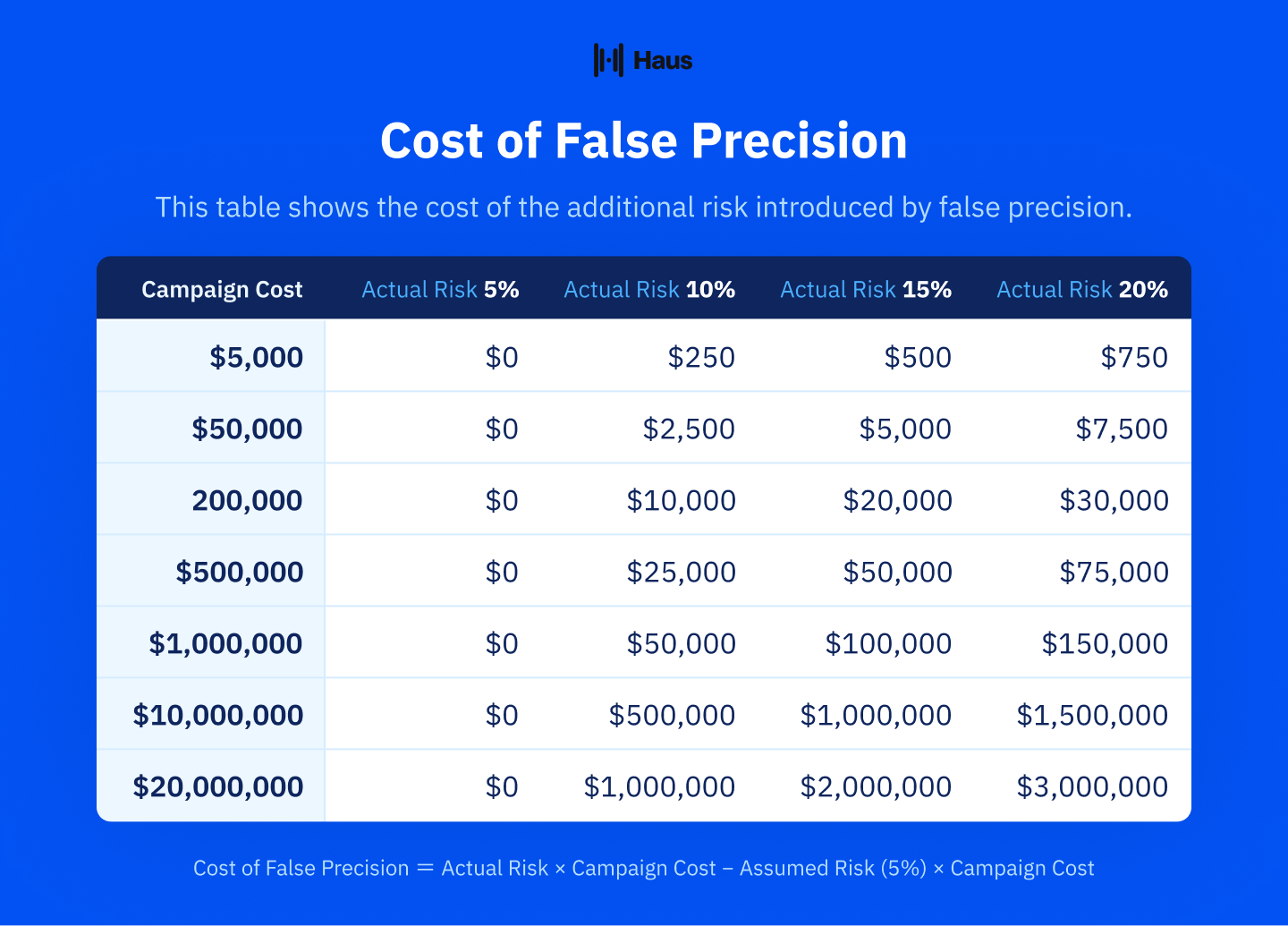
The Cost of False Precision formula quantifies the financial impact of misjudging risk in a test result. It compares actual risk to assumed risk and adjusts for probability to highlight the potential cost difference.
Let’s step into a hypothetical scenario: You’re spending $10M on a campaign and run a holdout experiment to see if it’s truly incremental. Based on the reported precision of the test, you determine that you are only taking a 5% risk of falsely detecting lift. That sounds pretty reliable, so you move forward.
But what if the experiment isn’t as precise as you thought? Imagine the actual chance of mistakenly detecting lift is 25%, not 5%. That would mean you’re only 75% confident in your results — not as reassuring!
Now, let’s say your $10M test falsely identifies lift when there is none. You end up pouring millions into a marketing campaign that drives no real impact. Would you be willing to risk that kind of waste with a 1-in-4 chance of being wrong? It’s like playing Russian roulette with your ad budget. Are the odds still worth it?
So… how do I know if I can trust my incrementality tests?
This is the (multi) million dollar question. When vetting incrementality partners, here are a few essentials we recommend keeping in mind related to precision, confidence, and trust:
- Bring a data scientist or someone with a background in causal inference to the conversation: Can your incrementality partner share information about standard errors? Can they tell you about the methodologies they use, like difference in differences, difference in means, or synthetic control? Your data science partner will be able to help you out here — see if you can even get your hands on standard error and confidence intervals that they can help evaluate.
- Ask for transparency around test power and standard error estimation: In both the design and analysis phase, it is critical to understand test power and standard error estimation. In the design phase, this understanding will help you gauge whether you’re spending enough money to detect lift and come out with a result you feel confident in. In the analysis phase, it will help you decide how much to bet on any given result. (And no, vague confidence scores or precision ‘green-lights’ are not enough.)
- Understand the assumptions of the measurement model: When things rest on a litany of assumptions but have a highly precise estimate, that’s a signal to be wary. Try to think about it rationally.
- Think about the implications: If your incrementality test results are suggesting you do something insane — like 10x your budget in a channel, for example — pause. Recommending a dramatic change can be a sign that the false certainty monster is afoot.
- Frustrating results aren’t necessarily a bad thing: If an incrementality partner is delivering you a disappointing result, that might actually be a good thing — it can be a sign they’re being honest and not sugarcoating. This is the person who’s telling you that you have salad in your teeth — you’ll want to thank them later.
- Trust your gut: We know this is a piece about science, but hear us out — if it feels too good to be true, it might be. Instincts exist for a reason.
There’s no shortcut for doing your homework. Precision matters, but guaranteeing a level of precision isn’t the goal of incrementality experiments — the goal is being honest about uncertainty so you can make better, more profitable decisions.