Why An Econometrics PhD Left Meta To Tackle Big Causal Questions at Haus
May 2, 2025
Before joining Haus as Senior Applied Scientist, Ittai Shacham was a senior researcher at Meta, where he built some of the company’s most sophisticated internal causal modeling tools. He sat down with us to talk about his path to Haus, life on the Haus Science team, and the diverse expertise needed to build robust causal models.
⏪ After a PhD in econometrics, you spent three years as a senior researcher at Meta. What did you work on there?
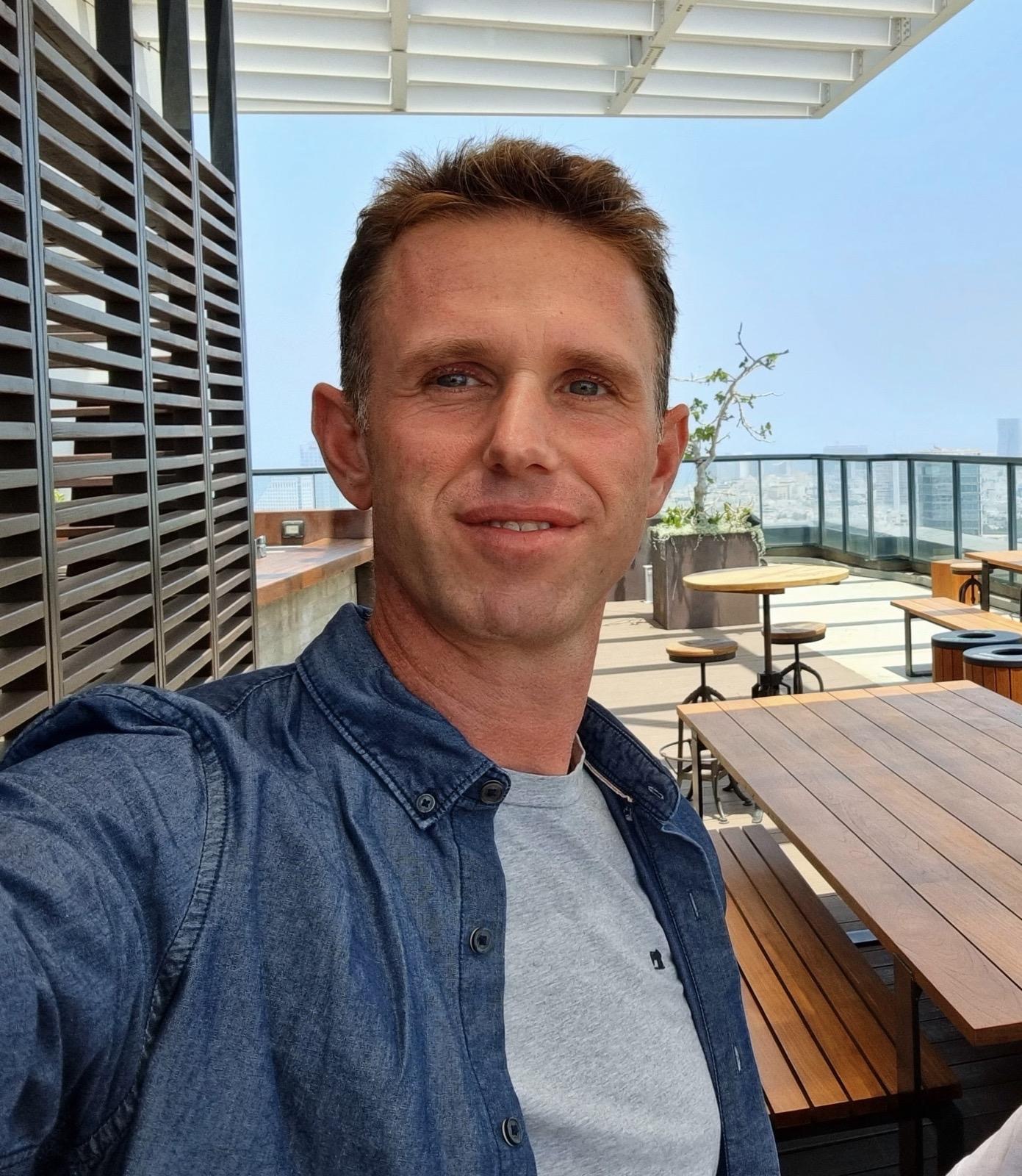
My last project at Meta was building a tool that helped solve the problem of continuous causal measurement. So let’s say you’re a brand. You need to know your current standing all the time in order to make informed decisions in real time. You aren’t going to run an experiment all the time, but you need some sort of continuous signal on ad performance.
You could think of it as similar to stock trading. The tool helps you figure out at what point the incremental value of spend on some ad campaign is below some threshold — then you sell.
We wanted to automate and optimize the entire process of advertising on Meta platforms. And so, the question was, “How could we create a continuous measure of incremental returns?”
So we combined experiments with ongoing data, using experiments that happened once in a while to tune an observational causality model. With scope and more clients using it, we gained continuous measurement of incremental value for ads. It opened up all these new avenues for the whole organization.
🏡 Then you came to Haus. What’s it like doing science at Haus?
It’s so enjoyable — it's a lot like working in an econometrics department at a university.
We have very good econometricians, very good machine learning experts, as well as experts from other scientific fields. And I love that we all bring our knowledge from other fields and combine them here.
For what we’re trying to do, we need a combination of talents and methods. It's not going to be pure econometrics — we also need to learn how to incorporate machine learning and deep learning methods with causality. All of these things will come together to create a model that’s just way more robust.
🤝 What does collaboration look like when you have so many diverse talents working together?
Well, you must remember that modeling, specifically causal modelling, is an art and a science. So I love econometrics because of its ambiguity – at the end of the day, it requires a leap of faith. You see a causal relationship, you go out and grasp it… but then it’s just smoke. Poof. It’s gone. That’s because it’s not always measurable. We must rely on implicit equations that capture the essence of the causal relationship, called exclusion restrictions.
So that’s where collaboration with machine learning experts comes in. Because, in contrast to us, the problems they tackle have clear, measurable outcomes. They can measure how well their models perform. So naturally, you can see how these two types of expertise fit together.
📏 Plus, you guys are trying to measure the most “un-measurable” thing: human behavior. That can’t be easy, can it?
Sure, people are fickle. People are irrational. People are emotional. There's a behavioral economist from Duke named Dan Ariely who coined the phrase “predictably irrational.” His idea: People are irrational, but they are irrational in very specific forms that are actually predictable. A finance economist from Stanford exemplified this using data from credit card providers in the US.
Plus, other behavioral economists and game theorists have studied emotions and their role in human evolution. They found that many of the emotions we consider “irrational” — like anger, for instance — create a beneficial equilibrium. There’s a structure to them.
So we can model human behavior because our irrationalities are structured. Plus, we must remember that a model is a gross simplification of reality. We're not trying to capture exact reality. We're trying to capture a very specific slice of it.
So while we’re after something very ambitious, it’s possible. And as we get closer to answers… well, that’s when things start to get really exciting.